Thank you for registering for our series. Keep an eye on your email for Article 4 – releasing in 2025.
No matter what type of data you’re collecting in an infrastructure project, you’ll almost always encounter one common hurdle — disparate data.
Data is essential for every project phase, but managing diverse datasets that vary in format, accuracy, and spatial coverage presents huge challenges for engineers. Taming this disparate data is key to making well-informed decisions and creating better outcomes for your projects.
In our previous article, we showed when ground engineering teams should choose 3D modelling, and how combining 1D, 2D, and 3D modelling helps provide better understanding and problem-solving in infrastructure projects.
In this article, we’ll look into the challenges of managing data that changes over time, as well as strategies and digital tools that make integration and real-time updates more manageable and efficient. Sharing their insights are:
- Fiamma Giovacchini, Customer Solutions Specialist at Seequent
- Peter Fair, 3D Ground Modelling Specialist at Mott MacDonald
- Jonas Weil, Geologist and Associate Partner at iC Group
What types of data do infrastructure projects deal with?
No matter what infrastructure project is underway, chances are you’ll encounter an enormous variety of different data types to handle — all of which are vital for informing your decisions and engineering strategies.
However, these data types are often disparate, meaning they’ll differ in structure, collection methods, spatial attributes, and temporal characteristics.
Understanding the different types of data that these projects typically handle — and what makes them disparate — is your first step towards managing and integrating them effectively throughout your project’s lifecycle.
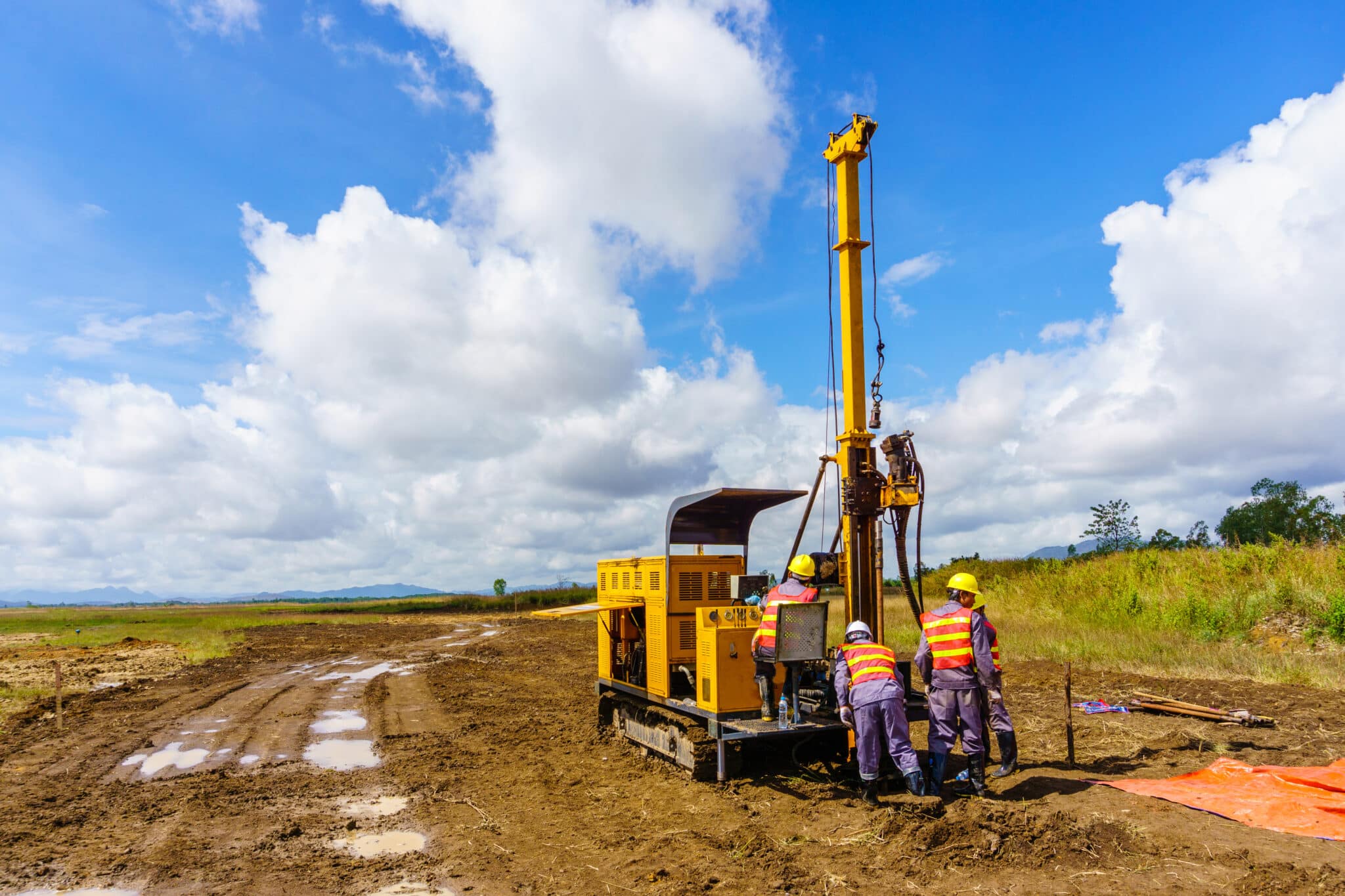
Soil sampling in the production of engineering-geological surveys.
Here are just some of the data types you’ll commonly encounter in engineering projects:
Geological
- Borehole logs
- Rock and soil samples
- Outcrop and face mapping
- Geological maps
- Structural measurements
- Cross-sections
Geotechnical
- In-situ testing, e.g. soil behaviour types from cone penetration tests
- Laboratory test results e.g. shear strength from triaxial testing
- Rock quality designation (RQD) from borehole logs
Environmental
- Gas & Groundwater monitoring and sample testing
- Environmental impact data such as contaminant contour maps
Geophysical
- Seismic refraction
- Resistivity profiles
- Magnetic susceptibility
- Ground- penetrating radar (GPR)
- Unexploded ordinance surveys
Geospatial
- Digital elevation models, Survey, Photogrametry, LiDAR, aerial imagery or satellite data
- Geographic Information Systems (GIS) data such as land use, Utilities, soil maps or property information (cadastre)
Engineering
- Plans and drawings
- Building Information models
- 3D engineering design models
What makes these types of data disparate?
- Structure – some data might be structured as points, lines, areas, volumes, or as 2D or 3D grids, resulting in a mismatch that makes analysis more challenging.
- Data collection methods – different instruments or techniques yield data in various resolutions. For example, seismic surveys produce waveforms, LiDAR generates point clouds, and borehole drilling provides detailed logs.
- File formats – how data is collected dictates the file formats used. Seismic data, LiDAR outputs, or borehole records often arrive in distinct, incompatible formats, which can complicate how data is integrated into datasets and significantly slow down the transformation of data into information.
“You might receive data with different resolutions or formats. Some might be very old, and therefore it might comply with different standards. And others might be low quality, conflicting, or obsolete by today’s standards.”
Peter Fair3D Ground Modelling Specialist, Mott MacDonald
- Spatial coverage – seismic surveys and LiDAR can cover large areas, while borehole data is relatively sparse, with data points typically spread over huge distances. Combining broad, continuous datasets with highly localised discrete data is complicated because of the variations in density and coverage.
- Positional accuracy – this depends on the collection method and instrumentation. For example, GPS-aligned LiDAR will be more accurate in terms of positioning than borehole data collected using less precise location markers. Any misalignments then create discrepancies when integrating datasets.
- Temporal variability – datasets are often collected at different stages of a project. Early-stage seismic data, for instance, might be collected several months or even years earlier than borehole sampling data, meaning data points may no longer represent the same conditions in a rapidly evolving environment.
“We frequently receive data from different subcontractors and investigation campaigns. That could be measurements of geomechanical parameters with different test methods, on different scales, with different resolutions and scatter.”
Jonas WeilGeologist and Associate Partner, iC Group
What challenges arise from disparate datasets?
Disparate data brings with it difficult challenges for geotechnical engineers — especially when project teams are trying to consolidate different datasets into a single, usable model.
One of the main challenges associated with disparate datasets is integration issues. Combining data from multiple sources into a unified model is time intensive and susceptible to errors. Incompatible data formats, spatial resolutions, and accuracy levels all need extra processing, and any misalignment can distort the final model.
Another challenge comes from visualising disparate data. Borehole data, for example, is typically vertical and discrete, while geophysical surveys like seismic data are 2D or 3D and continuous. Aligning these differing data types to show a realistic representation of subsurface conditions is incredibly difficult without the right tools.
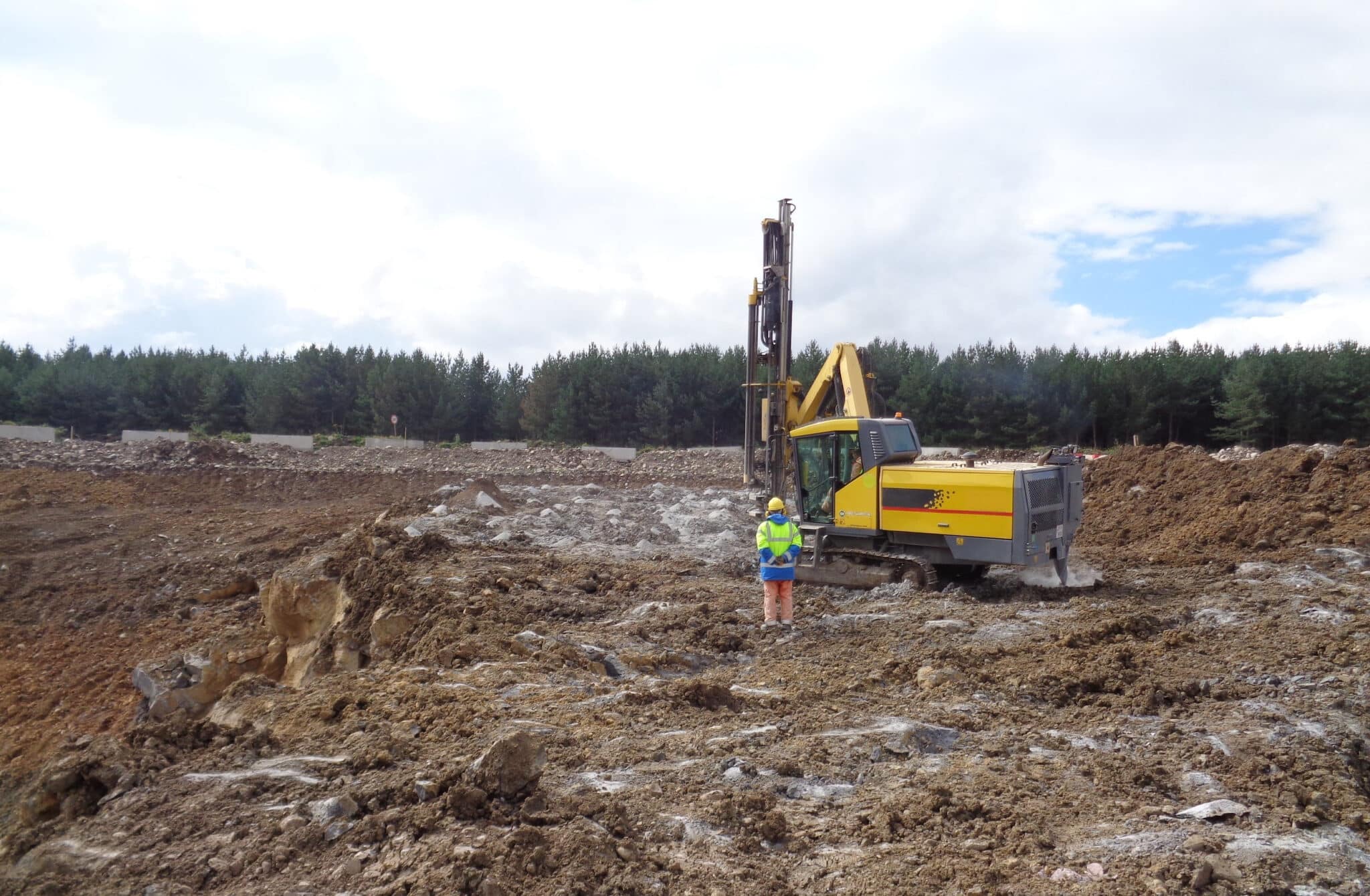
Hydraulic drill works during a highway / road construction project
The fourth dimension: How does data change over time?
Data doesn’t remain static — it evolves as projects progress, with each phase introducing new information and complexities.
Early project phases, such as planning and pre-tendering, often have limited data. However, as projects move into detailed design and tendering phases, data volumes increase almost exponentially.
Additional survey, testing, and remote sensing campaigns — like new boreholes or LiDAR studies — all bring in fresh insights that cause the dataset to evolve.
This accumulation of data, which is continuously being updated and refined over time, has its challenges. While continuous updates help to maintain an accurate, real-time model, it can also become unwieldy without the right data management tools.
“Without the right tools, you can encounter huge challenges. This data comes from different times, so for full traceability and auditability, we need to know what was produced when.”
Fiamma GiovacchiniCustomer Solutions Specialist, Seequent
Case study: The HS2 rail project
Large-scale projects, such as the HS2 rail project in the UK, show how data evolves over time. New borehole data, environmental assessments, and other studies were continuously added throughout the project’s lifespan, updating the model regularly with real-time findings.
Rather than one single, static dataset, the project model became a dynamic, iterative resource that adapted as fresh information was added.
Handling the temporal dimension of data calls for an agile approach, where new data can be integrated seamlessly. This allows teams to keep models up to date, refine designs, and respond to evolving project demands while maintaining a comprehensive overview of subsurface conditions that spans the entire project’s timeline.
Do guidelines exist for handling this type of data?
Guidelines for managing and using site investigation data are essential for consistency, quality, and compliance across projects, especially when dealing with complex, evolving datasets. These guidelines range from internationally recognised frameworks to country-specific standards and even best practices within individual companies.
International guidelines
International standards create a broad framework that can be adapted across different project types and regions, helping to make geotechnical data management more cohesive.
For example, in 2023, the International Association for Engineering Geology and the Environment (IAEG) released guidelines for the development and application of engineering geological models — a comprehensive knowledge framework for the consideration of all the geological conditions and their engineering characteristics relevant to a project. The guidelines are designed to provide practical advice on the effective use of engineering geological models for a wide range of applications including civil engineering.
Country-specific guidelines
As well as international standards, individual countries have developed specific guidelines tailored to their own regulatory and environmental standards. These guidelines impact how data is collected, stored, and exchanged in geotechnical projects within each country.
In the UK, for instance, organisations like the Association of Geotechnical and Geoenvironmental Specialists (AGS) and projects like DIGGS in the US provide standards to help engineers streamline how they exchange data and keep it compatible across different platforms and tools.
Company-specific best practices
Many organisations and companies in the infrastructure sector also develop their own internal guidelines, especially when working across multiple countries with varying regulations.
These company-specific practices help standardise data management while still aligning with international frameworks and local guidelines to adapt with the demands of each project location.
Public organisations and infrastructure owners are also becoming more and more aware that underground information is an asset.
“Large organisations have their internal regulations for including geological data in their databases. One of our clients handles a borehole register of over 55,000 borehole profiles from very different sources. This data has been collected across more than 100 years to be systematically interpreted when building new infrastructure, like the metro extension.”
Jonas WeiliC Group
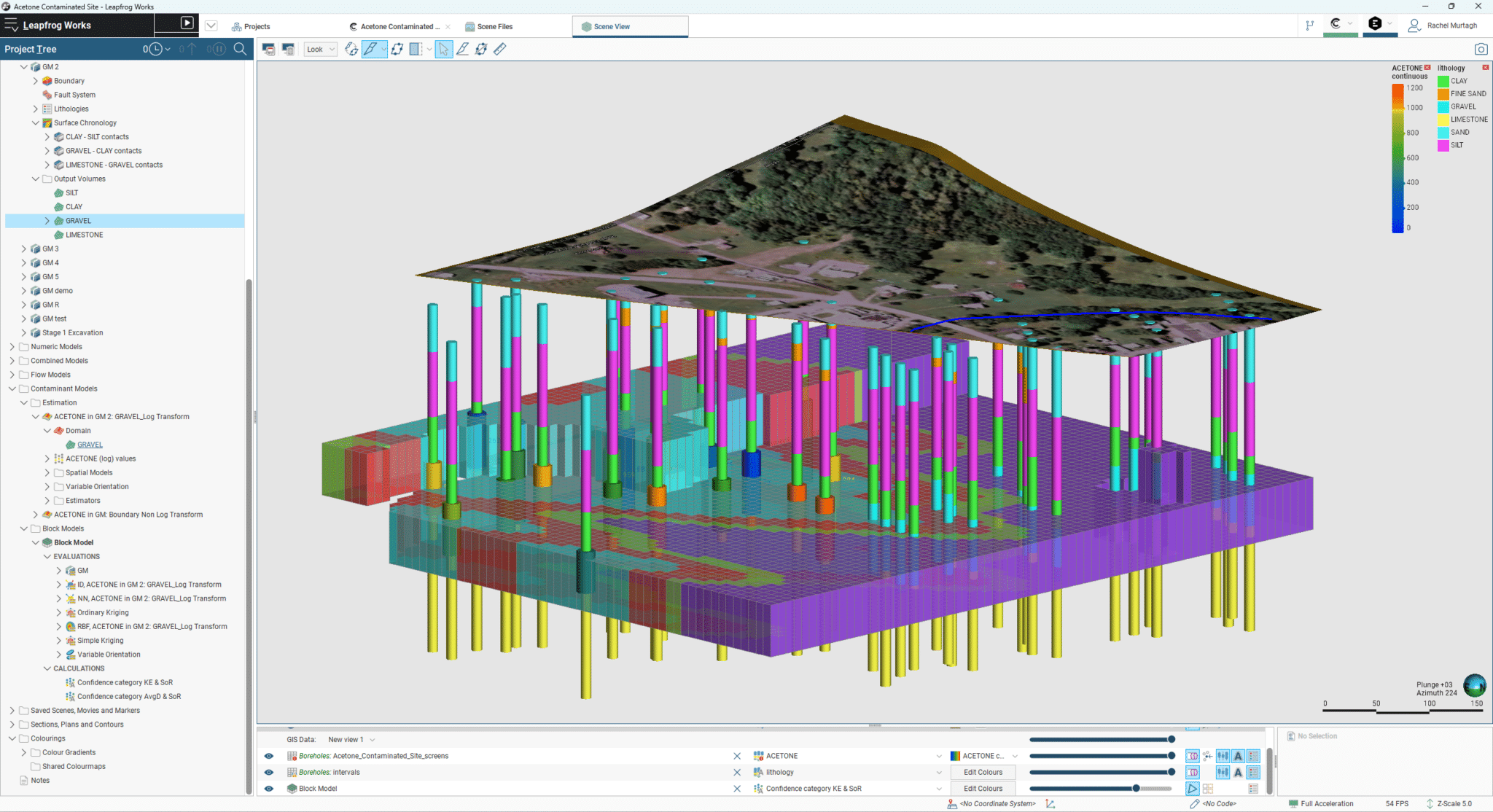
Seequent’s Leapfrog Works used to create a geostatistical model of estimated acetone levels within a contaminant plume.
How can digital tools make managing disparate data easier?
When handling a myriad of disparate datasets — which can vary in type, format, and source — digital tools provide an easier way to integrate, visualise, and manage data.
3D visualisation tools have transformed how engineers interact with subsurface data. CAD-based programs, for example, let teams manipulate complex datasets into visual formats, allowing them to create detailed design models for engineered structures. However, these programs often fall short when it comes to working with subsurface data to build engineering geology models. Tools like Leapfrog Works offer 3D modelling options specifically designed for subsurface data, making it easier for engineers to integrate data from multiple sources to more rapidly understand site conditions and risks.
Seequent’s suite of geotechnical tools, including OpenGround, Central, GeoStudio, and PLAXIS, is geared towards managing and integrating diverse geotechnical data. By supporting multiple industry-standard formats, they make it easier than ever to align with international and country-specific regulations.
Increasing productivity
Digital tools help to make data flow more seamless, with dynamic updates between software platforms to make sure every project component is up to date with the latest data.
Cutting out the repetitive, manual tasks — like reformatting data and updating models — then frees up time for more valuable work, such as hypothesis testing and informed decision-making. This ultimately reduces project delays and allows engineers to make faster, more informed decisions that could spell the difference between project failure and success.
“Going through those iterative phases of a project is much faster, much less time-consuming with digital tools. What used to take weeks is now done in hours.”
Fiamma GiovacchiniSeequent
By allowing different teams to access shared data and models simultaneously, digital tools also support real-time collaboration, improving communication and ensuring teams always have the latest project information to hand.
Reducing errors
Digital tools also help reduce errors by improving data accuracy and quality control. Automated integration and quality control mechanisms minimise human error in data handling, and the ability to regularly update a dynamic model with new data ensures that data is always up to date and reliable.
Visualisation platforms like Leapfrog help users explore disparate data types within a unified 3D environment, providing a better understanding to spot potential errors early on, before they can impact the design.
“Leapfrog is really good at handling different densities of data. The proximity to measured data is one of the keys to understanding your uncertainty.”
Fiamma GiovacchiniSeequent
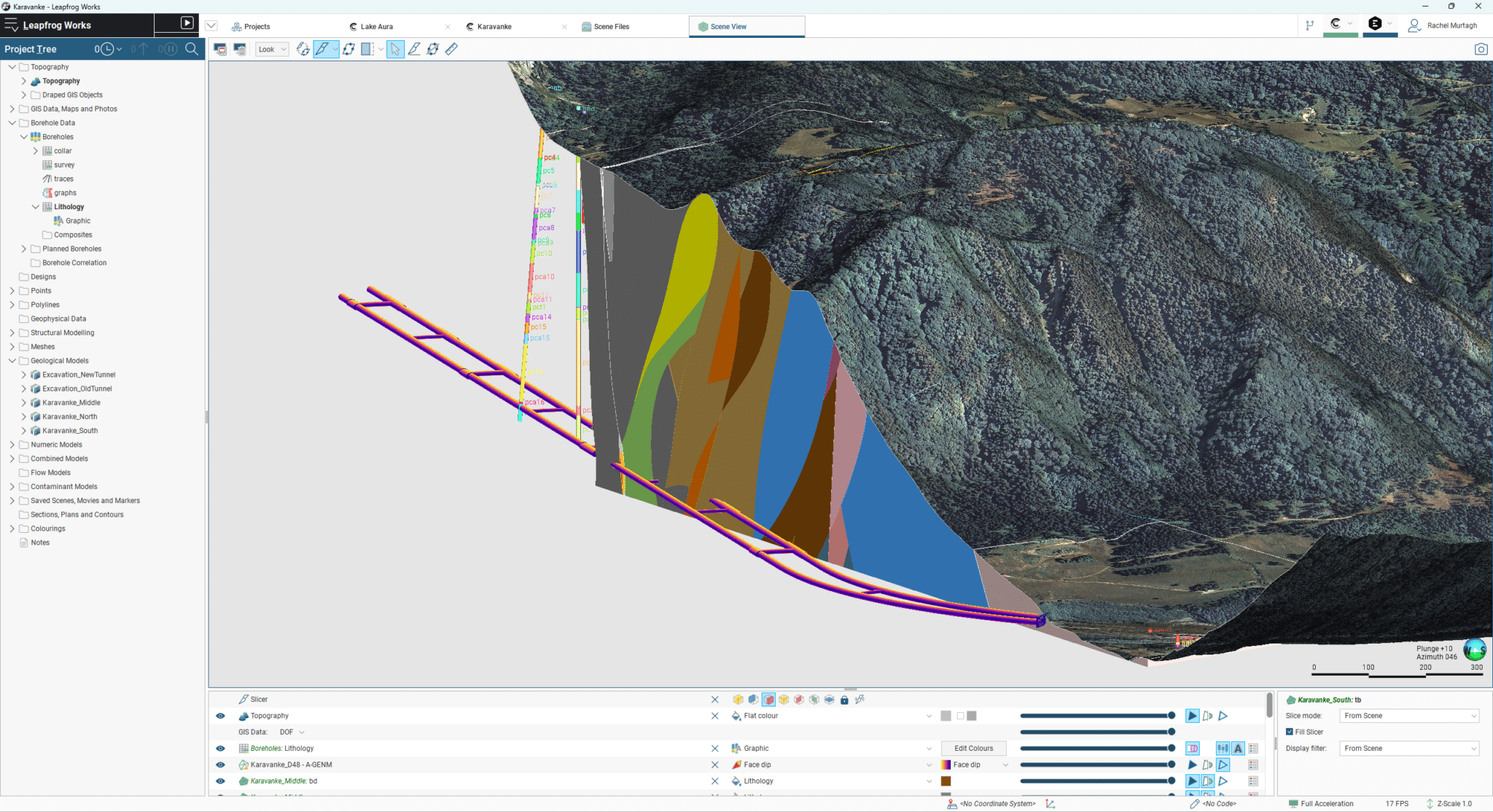
A complex series of faulting along a new parallel tunnel route. Created within Seequent’s Leapfrog Works from limited borehole data, relying on historic face maps from the original tunnel.
Auditability features in digital tools also help teams track data changes over time, allowing full traceability and transparency when making decisions. This tracking helps teams to manage uncertainty and lead to less conservative designs, potentially creating savings on project costs.
“On a project level, digital tools help reduce risk by bringing together all available information to create a single source of truth. They give us the ability to visualise otherwise complex and technical data in a more accessible way.”
Peter FairMott MacDonald
From disparate data to actionable insights
In infrastructure projects, managing data that is diverse, expanding, and varied across project phases can be a complex task for engineers. Differences in data formats, accuracy, and spatial coverage cause challenges with integrating data that can impact project timelines and outcomes.
Tools like Seequent’s are designed to simplify this integration process, allowing seamless, dynamic integration to boost productivity while also reducing errors. Through real-time visualisation, auditability, and quality control, digital tools transform disparate data into actionable insights.
Reliable, iterative workflows help engineering teams make well-informed decisions, ensuring that every phase of a project is grounded in accurate, reliable, and up-to-date information.