Esta es una revisión técnica de la herramienta Interpolador RBF, destinada a lograr flujos de trabajo robustos y dinámicos en su modelado numérico.
We will take a deeper look at how Leapfrog Geo constructs a numerical model in order to build upon and provide a better understanding of the tools available at your disposal.
Duración
49 min
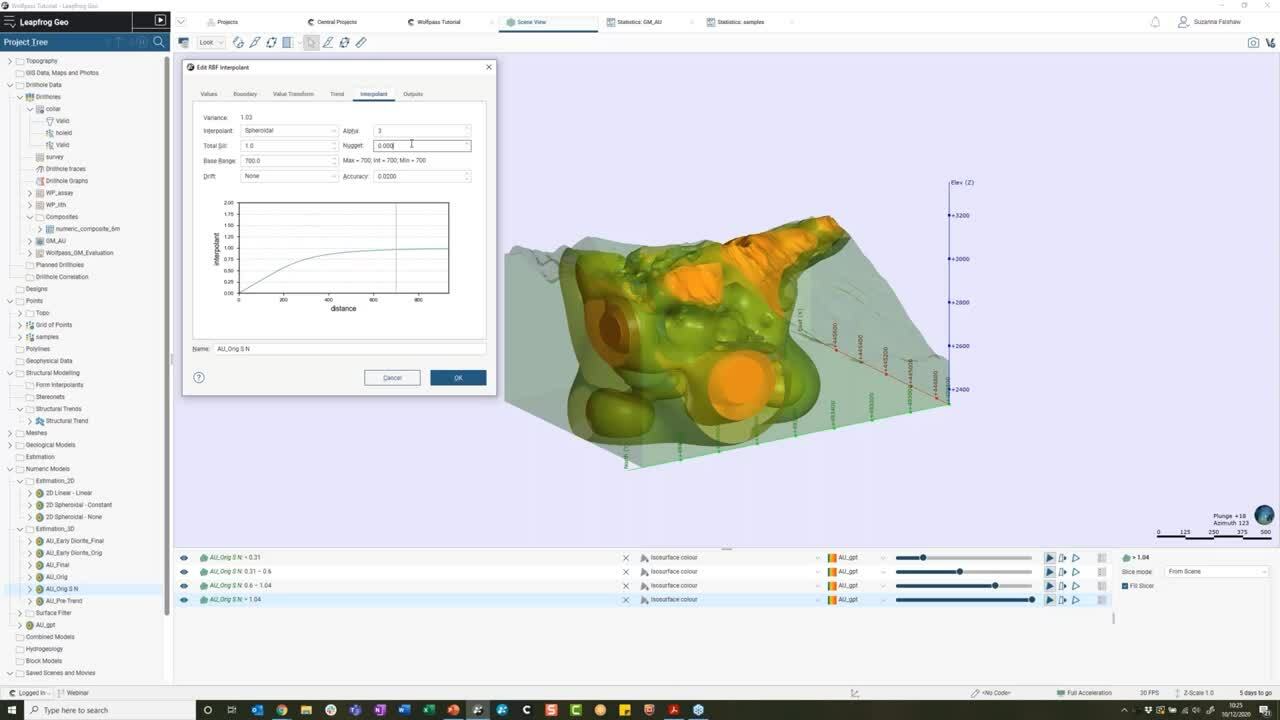
Ver más videos bajo demanda.
VideosObtenga más información acerca de la solución para la industria minera de Seequent.
Más informaciónVideo Transcript
[00:00:11.105]
<v Suzzana>All right, hello and welcome everyone.</v>
[00:00:14.420]
I make that 10 o’clock here in the UK.
[00:00:16.324]
And so, let’s get started.
[00:00:19.231]
Thank you for joining us today for this tech talk
[00:00:23.050]
on Numeric Modeling elite for GA.
[00:00:26.373]
By way of introduction, my name is Suzanna.
[00:00:29.290]
I’m a Geologist by background based out of our UK office.
[00:00:33.624]
And with me today are my colleagues,
[00:00:36.510]
James and Andre both of him will be on hand to help
[00:00:39.930]
run this session.
[00:00:41.658]
Now, I run for this session is
[00:00:44.680]
to refocus in on the interplant settings
[00:00:48.204]
that will most effectively improve your numeric models
[00:00:52.240]
from the word go.
[00:00:54.320]
Let me set the scene for my project today.
[00:00:56.820]
This is a copper gold porphyry deposit.
[00:00:59.817]
And from the stats,
[00:01:01.325]
we know that it is our early diorite unit
[00:01:06.920]
that is a highest grade domain.
[00:01:10.560]
For the purpose of today’s session,
[00:01:13.220]
I’m going to focus on modeling the gold asset information,
[00:01:17.081]
Some port from these drill holes, but in reality,
[00:01:20.650]
I could choose to model any type of numerical value here,
[00:01:26.320]
for example, our QT data.
[00:01:29.010]
And if that is the workflow that you’re interested in,
[00:01:31.160]
then I’d recommend you head over to the secret website,
[00:01:34.197]
where there were a couple of videos
[00:01:36.560]
on this topic already available.
[00:01:40.000]
So coming down to my numeric models folder,
[00:01:44.520]
I’m now going to select a new RBF interplant.
[00:01:51.500]
And in the first window,
[00:01:53.840]
start to specify my initial inputs and outputs.
[00:01:59.310]
First, I’m going to want to specify
[00:02:02.130]
some suitable numeric values.
[00:02:04.440]
So in this case,
[00:02:05.720]
I’m going to use my composited gold ISO table,
[00:02:10.407]
but I can also choose to apply any applicable query filter.
[00:02:15.800]
Now, in this case, as part of my initial validation steps,
[00:02:20.658]
I actually created a new column in my collar table
[00:02:25.847]
called valid simply to help me identify which drill holes
[00:02:32.300]
I want to use in my modeling.
[00:02:35.017]
I did this by first of all,
[00:02:37.380]
creating a new category selection
[00:02:40.640]
to make those initial selections
[00:02:43.320]
then created a very simple query filter
[00:02:46.776]
simply to exclude any of those that are invalid.
[00:02:51.900]
And actually for the most part,
[00:02:53.830]
it’s just this one hole at the bottom
[00:02:57.290]
that I chose to exclude.
[00:03:00.960]
As I work on my interpretation or indeed bring in more data,
[00:03:04.416]
then it’s always useful to have flexibility
[00:03:07.000]
like this built into your drill hole management workflow.
[00:03:10.310]
So I would encourage you to set up something similar
[00:03:13.580]
if not already done so,
[00:03:16.189]
but for now though, let me just go back to my,
[00:03:20.660]
I’ll be up in turbulent definition again,
[00:03:25.791]
and let’s start to talk about the interplant boundary.
[00:03:32.950]
Now, the default here is set to be our clipping boundary.
[00:03:40.850]
And if you don’t know what that is,
[00:03:42.660]
or if you want to set that,
[00:03:43.700]
then you can actually do that from either the topography
[00:03:46.130]
or indeed the GIS data folder.
[00:03:49.950]
So I could choose to manually change this boundary
[00:03:54.360]
or to enclose it around any object
[00:03:58.360]
that already exists in my project,
[00:03:59.920]
or indeed an existing model boundary or volume.
[00:04:05.960]
Whenever I set here in my interpret boundary
[00:04:09.950]
is going to be linked to the surface filter
[00:04:13.788]
or by default is linked to the surface filter,
[00:04:17.350]
which in itself controls which values can go into the model.
[00:04:22.920]
I’m just going to bring a quick slice view into my scene,
[00:04:27.220]
just to sort of help us visualize
[00:04:28.955]
what I’m about to talk about.
[00:04:32.020]
But I will explain this as this space
[00:04:33.180]
is just a slice of my geological model.
[00:04:36.531]
Just with those sort of gold values seen in a scene here.
[00:04:43.120]
Typically your interplant boundary
[00:04:45.960]
would be set to enclose the entire data set
[00:04:51.490]
in which case all of your specified input values
[00:04:56.150]
will become interpolated.
[00:04:57.570]
So that’s kind of what we’re seeing in scene at the moment.
[00:05:00.150]
It’s just a simple view of everything
[00:05:02.975]
that’s in my data and in my project,
[00:05:08.380]
I could choose however,
[00:05:09.746]
to set a boundary around an existing volume.
[00:05:14.380]
So for example,
[00:05:15.320]
if I want to just specifically look at my only diorite
[00:05:18.320]
Which is anything here in green,
[00:05:20.830]
then I can choose to do that.
[00:05:23.550]
And if I just turn that on now, then you can see,
[00:05:28.950]
we’re just seeing that limited subset
[00:05:32.090]
of input values in that case.
[00:05:35.187]
Now, interestingly, if I wanted to add this point,
[00:05:39.230]
mimic a soft boundary around the early diorite
[00:05:42.900]
So for example, some sort of value buffer
[00:05:47.180]
say it’s about 50 meters away,
[00:05:50.800]
which is this orange line here.
[00:05:53.700]
Then I could incorporate this also into my surface filter.
[00:06:00.300]
And again, by doing, say,
[00:06:02.240]
so if I now select this distance function here,
[00:06:08.260]
then again, we’re going to see this update
[00:06:12.270]
on which input values can be used into the model.
[00:06:17.610]
But now though let’s not complicate things too much.
[00:06:20.610]
I’m just simply going to use the same boundary
[00:06:25.330]
as my geological model.
[00:06:28.870]
And I’m also going to bring down my surface resolution
[00:06:33.196]
to something a bit more reasonable.
[00:06:35.630]
Now, a rule of thumb with drill hole data would be
[00:06:39.580]
to set this to your composite length.
[00:06:42.690]
So as to equal the triangulation
[00:06:45.752]
or indeed some multiple of this,
[00:06:48.980]
if you find the processing time takes too much of a hit
[00:06:52.752]
for this particular data set,
[00:06:55.360]
I have six meter lens composites already defined.
[00:06:59.169]
So I’m going to bring surface ISO down
[00:07:02.800]
to multiple of that 12 in this case.
[00:07:06.490]
And of course those composites are there
[00:07:08.660]
in order to help normalize
[00:07:10.400]
and reduce the variance of my data.
[00:07:13.870]
If I didn’t already have numeric complicit set up
[00:07:18.770]
in my drill hole data folder,
[00:07:21.186]
then I could actually go into find these here
[00:07:25.770]
as well directly.
[00:07:28.608]
But now though, let me just go back and use those,
[00:07:31.840]
these composites that I have.
[00:07:35.180]
And for now, let’s just say, okay to this and let it run.
[00:07:44.920]
All right, now, there will be some times here
[00:07:47.884]
that it’s going to be easier just to jump into models
[00:07:51.179]
that have already been set up.
[00:07:52.817]
And I think this is one of those cases.
[00:07:54.940]
So let me just bring in that is going to generate,
[00:08:02.120]
which is essentially our first pass gold numeric model.
[00:08:09.980]
And if I stick the legend on,
[00:08:12.330]
then you’ll start to get idea
[00:08:14.030]
or reference point for that grade.
[00:08:17.390]
Now, the power of leap frogs on behalf energy engine
[00:08:21.680]
is in its ability to estimate a quantity
[00:08:25.780]
into an unknown point by using known drill hole
[00:08:29.760]
or point data.
[00:08:31.830]
It’s important however,
[00:08:33.540]
when we estimate those unknown points
[00:08:36.770]
that we’re selecting an interpolation function,
[00:08:40.383]
that will make the most geological sense
[00:08:44.730]
at this first pass stage.
[00:08:47.220]
I hope you’ll agree that we’re seeing something
[00:08:49.732]
that’s very unrealistic,
[00:08:52.110]
especially in regards to this higher grade sort of blow outs
[00:08:56.520]
in my Northwest corner.
[00:09:00.680]
Is fairly common for our projects
[00:09:03.970]
to have some areas of data scarcity.
[00:09:07.320]
If I bring my drill holes in here
[00:09:11.086]
and just turn the filter off for a second,
[00:09:17.330]
then I’m sure you agree that that sometimes at depth
[00:09:21.639]
or indeed on our last full extents,
[00:09:25.280]
that we might just not have any drilling information
[00:09:29.060]
in that area.
[00:09:31.740]
And in this case,
[00:09:32.960]
it is the, it’s just three drill holes here.
[00:09:36.852]
If I put my filter on,
[00:09:42.370]
you can see that it’s just these three drill holes
[00:09:45.241]
that is simply causing that interpolation here
[00:09:49.640]
to become really quite extrapolated.
[00:09:53.340]
And unfortunately there’s many an extreme example in line
[00:09:56.620]
of such models.
[00:09:57.660]
The C’s finding their way into company reporting.
[00:10:02.220]
So what I would encourage you all to do,
[00:10:04.890]
is to of course start refining the internal structure
[00:10:07.890]
of my model and the majority of the functionality
[00:10:11.290]
to do so actually sits under the interplant tab.
[00:10:16.590]
So if I go to the model
[00:10:17.640]
and I’ll go to the one that’s run individually,
[00:10:22.520]
so just double click into it
[00:10:24.260]
and I’m going to come to the interplant tab.
[00:10:29.150]
For now, I’m simply going to change my interplant type
[00:10:34.640]
to a splital and change my drift function to known
[00:10:41.240]
I will come back to this in more detail, but for now,
[00:10:43.720]
let me just let that run and we’ll have a look
[00:10:47.380]
at what that produces instead.
[00:10:50.960]
And again, great.
[00:10:52.220]
Here’s one I prepared earlier,
[00:10:58.550]
which we can now see,
[00:11:00.910]
and hopefully you can already see
[00:11:02.800]
this big notable difference already,
[00:11:05.630]
especially where a high grade
[00:11:10.130]
or where that original high-grade was blown out.
[00:11:15.180]
This time, if I bring my drill holes back in again,
[00:11:21.870]
we can see that high grade interpolation
[00:11:25.677]
around those three drill hole still exists,
[00:11:29.310]
but just with a much smaller range of influence,
[00:11:34.040]
but why is that the case to help answer that question,
[00:11:38.250]
I’m going to try and replicate these RBF and parameters
[00:11:42.030]
on a simple 2D grid of points.
[00:11:45.290]
And this should quite literally help connect the dots
[00:11:49.850]
in our 3D picture.
[00:11:52.020]
So let me move from this for a second
[00:11:54.410]
and just bring in my grid of points
[00:11:59.070]
and a couple of arbitrary samples.
[00:12:02.760]
Hopefully you can see those values starting to come through.
[00:12:11.340]
Here we go.
[00:12:14.520]
So what I’ve done so far for this is,
[00:12:17.820]
I have created three new RBF models
[00:12:24.040]
in order to estimate these six points shown on screen here.
[00:12:29.140]
And then I will come back into each of the Interplant tabs
[00:12:34.728]
in order to adjust the interplant interests settings.
[00:12:41.600]
And that’s just what the naming refers to here.
[00:12:47.380]
Now, leap for geezers two main interplant functions,
[00:12:52.670]
which in very simple terms will produce different estimates
[00:12:57.530]
depending on whether the distance
[00:12:59.190]
from our known sample points,
[00:13:01.308]
the range is taken into consideration.
[00:13:06.019]
And linear interplant will simply assume
[00:13:10.360]
that any known values closer to the points
[00:13:13.840]
you wish to estimate
[00:13:15.550]
we’ll have a proportionally greater influence
[00:13:18.610]
than any of those further away.
[00:13:22.040]
A splital interplant on the other hand
[00:13:25.480]
assumes that there is a finite range or limit
[00:13:30.160]
to the influence of our known data
[00:13:32.700]
beyond which this should fall to zero.
[00:13:36.141]
You may recognize the resemblance here
[00:13:39.130]
to a spherical very grim,
[00:13:42.060]
and for the vast majority of metallic ore deposits,
[00:13:45.960]
this interpolation type has more applicable
[00:13:49.430]
exceptions to this, maybe any laterally extensive deposit
[00:13:53.240]
like coal or banded iron formations.
[00:13:57.670]
So in addition to considering the interpolation method,
[00:14:00.870]
we must always also decide how best to control
[00:14:05.510]
our estimation in the absence of any data.
[00:14:09.308]
In other words,
[00:14:10.680]
how should our estimation behave
[00:14:13.510]
when we’re past the range of our samples,
[00:14:17.490]
say in this scenario we saw just a minute ago
[00:14:20.540]
with these three drill holes,
[00:14:23.610]
but this, we need to start defining an appropriate drift
[00:14:27.140]
from the options available.
[00:14:29.240]
And I think that was the point that I sort of got into
[00:14:33.300]
looking at on my grid.
[00:14:35.340]
So at the moment I have a linear interplant type
[00:14:40.670]
with a linear drift shown
[00:14:43.520]
and much kind of like the continuous column bludgeoned
[00:14:46.890]
than I have up here,
[00:14:48.470]
we’re seeing a linear,
[00:14:49.560]
a steady linear trend in our estimation.
[00:14:54.130]
The issue is, it was the estimation around
[00:14:57.660]
our known data points is as expected.
[00:15:00.497]
The linear drift will enable values
[00:15:03.690]
to both increase past the highest grade.
[00:15:08.420]
So in this case,
[00:15:09.253]
we’re sort of upwards to about a grade of 13 here,
[00:15:13.937]
as well as go into the negatives past the lowest grade.
[00:15:21.700]
So for great data of this nature,
[00:15:25.260]
we’re going to want to reign that estimation in
[00:15:29.010]
and start to factor in a range of influence.
[00:15:34.190]
So looking now at our splital interplant
[00:15:37.931]
with a drift of known,
[00:15:41.130]
we can see how the range starts to have an influence
[00:15:44.430]
on our estimation and then when we move away
[00:15:48.820]
from our known values.
[00:15:50.780]
So for example, if I start to come out
[00:15:52.770]
onto the extents here, then the estimation is falling
[00:15:57.910]
or decaying back to zero.
[00:16:00.790]
And that will be the same
[00:16:02.180]
if I sort of go around any of these,
[00:16:05.000]
we would expect to be getting down to a value as zero
[00:16:09.338]
away from any data points.
[00:16:13.760]
And of course, if you are close to the data point,
[00:16:16.170]
we would expect it to be an estimation similar.
[00:16:21.700]
So where you’re modeling an unconstrained area,
[00:16:26.290]
or perhaps don’t have many low grade holes
[00:16:29.770]
to constrain your deposit,
[00:16:31.811]
using a drift of known will ensure
[00:16:34.890]
that you have some control on how far your samples
[00:16:38.630]
would have influenced.
[00:16:40.410]
That said, if you are trying to model something constraints,
[00:16:44.500]
for example, the early diorite domain that we saw earlier,
[00:16:49.450]
then using a drift function of constant
[00:16:54.850]
could be more applicable.
[00:16:56.588]
In this case, our values are reverting
[00:17:00.480]
to the approximate mean of the data.
[00:17:03.030]
So if I just bring up the statistics our mean here is 4.167,
[00:17:12.010]
which means that as I’m getting to the outskirts,
[00:17:14.910]
I would expect it to be coming back towards that mean.
[00:17:18.960]
So a few different options, but of course,
[00:17:22.440]
different scenarios in how we want to apply these.
[00:17:26.240]
Now, if I jump back now to my gold model,
[00:17:35.070]
then let’s start first of all,
[00:17:36.580]
just with a quick reminder of where we started,
[00:17:41.280]
which was with this model,
[00:17:44.170]
and this is applying that default linear interplant,
[00:17:49.530]
and that simply by changing this already
[00:17:54.030]
to they splital or interplant type
[00:17:58.360]
along with a drift of known,
[00:18:02.010]
then we’re starting to see something
[00:18:04.330]
that makes much more sense.
[00:18:08.791]
So if I go back now and go back to my interplant tab
[00:18:15.870]
to sort of look at some of these other settings.
[00:18:20.924]
So far we know we want to limit the influence
[00:18:27.030]
of our known data to a certain distance,
[00:18:30.820]
and that it’s the distance reign
[00:18:33.020]
essentially controlling that correlation.
[00:18:37.230]
It’s reasonable, therefore that you’re going to want to,
[00:18:40.110]
you’re going to want to change the base range
[00:18:42.610]
to something more appropriate.
[00:18:44.720]
And if you don’t know where to start,
[00:18:46.170]
then a rule of thumb could be around twice
[00:18:49.680]
the drill hole spacing.
[00:18:51.240]
So in this case, let’s, that’s around 700,
[00:18:55.170]
which is appropriate for this project.
[00:18:59.350]
We also want to consider our nuggets and in leapfrog,
[00:19:04.000]
this is expressed as a percentage to our some.
[00:19:09.070]
Increasing the value of the nugget will create smoother
[00:19:13.640]
results by limiting the effects of extreme outliers.
[00:19:18.660]
In other words, we would give more emphasis
[00:19:21.972]
to the average grades of our surrounding values
[00:19:26.190]
and less on the actual data point.
[00:19:29.490]
It can basically help to reduce noise
[00:19:32.330]
caused by these outliers
[00:19:34.630]
or with inaccurately measured samples.
[00:19:39.090]
What value to use here is very much decided on a deposit
[00:19:43.420]
by deposit case.
[00:19:44.780]
And by all means, if you or someone else
[00:19:48.500]
in your organization has already figured this out
[00:19:52.030]
for your deposit, then by all means apply it here,
[00:19:55.570]
perhaps for a gold deposit like this.
[00:19:58.970]
Then a rule of thumb will be,
[00:20:02.280]
let’s say, 20 to 30% of the sales.
[00:20:05.410]
So let’s just take this down.
[00:20:07.170]
For example, it’s 0.2, which is going to be 20% of that.
[00:20:10.793]
So I have 10% might be more appropriate
[00:20:14.170]
for other metallic deposits or indeed known,
[00:20:17.400]
if you have a very consistent data set,
[00:20:21.560]
we’ve spoken about drift already,
[00:20:25.220]
but now that we are pressed the range of influence,
[00:20:29.430]
then it’s really our drift that comes into play.
[00:20:33.520]
Using known in this case is going to help
[00:20:37.220]
control how far my samples will have an influence
[00:20:41.409]
before the estimation to case back to Siri.
[00:20:46.329]
I’m not going to delve too much
[00:20:50.140]
into the other settings here.
[00:20:51.850]
You can always read about these
[00:20:53.410]
to your heart’s content online,
[00:20:56.250]
but the take home point really is that,
[00:20:58.720]
it’s the interplant the base range,
[00:21:03.320]
that percentage nuggets to the sill and drift
[00:21:07.840]
that will have the most material effects
[00:21:10.480]
on your numeric model.
[00:21:13.400]
Get these rights and you should be well on your way
[00:21:16.030]
to producing a very best model
[00:21:17.970]
that makes the best of your geological knowledge.
[00:21:21.060]
All right, now we’ve talked a little bit about
[00:21:24.460]
these interplant settings.
[00:21:27.310]
I thought it would be worth a quick run over
[00:21:30.706]
on the ISO surfaces.
[00:21:33.750]
So we can see all these,
[00:21:35.860]
as we expand out our new model objects,
[00:21:39.530]
we’ve got ISO surfaces
[00:21:41.520]
and then of course the resulting output volumes.
[00:21:45.420]
When we pick cutoffs in our outputs tab,
[00:21:50.020]
we are simply asking leap frog to define some boundaries
[00:21:54.047]
and space and your contours,
[00:21:56.990]
where there is the same consistent grade.
[00:22:00.520]
Essentially the iso surfaces perform the exact same task
[00:22:05.910]
as our geological surfaces do
[00:22:08.696]
when we’re geologically modeling.
[00:22:10.776]
But instead of building these surfaces ourselves,
[00:22:14.690]
we’re simply picking grade boundaries
[00:22:17.051]
that we want to see and leapfrog we’ll go
[00:22:20.330]
and build them for us.
[00:22:23.250]
It is this contour surface that,
[00:22:26.343]
that we’re seeing here in 3D.
[00:22:29.770]
And if we want to visualize a specific value,
[00:22:34.020]
then we can do so by updating the ISO values here
[00:22:40.760]
before I do that, let me just jump back
[00:22:44.850]
into the grid of points,
[00:22:46.980]
just to highlight this on a sort of simpler dataset.
[00:22:53.947]
So back in with my grid of points, let’s say for instance,
[00:22:58.810]
I now want to visualize a contour surface
[00:23:03.350]
with a greater two, then I can replicate that here
[00:23:07.612]
simply by setting some appropriate value filters.
[00:23:12.049]
And we can see that now
[00:23:14.520]
sort of finding all of these sort of values of two in space.
[00:23:18.746]
And these it’s this point that of course
[00:23:22.840]
is going to become our contour.
[00:23:27.900]
For the hikers amongst us
[00:23:29.401]
who are maybe used to seeing elevation contour lines
[00:23:32.550]
on a TD map.
[00:23:33.850]
This is the same principle,
[00:23:35.492]
except in the case of our gold model.
[00:23:38.180]
We’re simply looking at a value representation in 3D.
[00:23:43.060]
So let me go back to my gold model once again
[00:23:49.690]
and actually set some better ISO values for this deposit.
[00:23:57.710]
So am just going to go double click back in
[00:23:59.960]
and head over to the output tab.
[00:24:02.870]
So let’s just set a few, make a little bit more sense
[00:24:06.670]
than the ones that are put in as default.
[00:24:10.900]
So let’s say 0.5, 0.75.
[00:24:16.350]
Let’s do 1.25 and at 1.5, I think we’ll suffice.
[00:24:27.970]
The other thing that I’m going to do
[00:24:29.640]
is take down the resolution on my higher grades
[00:24:33.990]
to try and be as accurate as possible.
[00:24:38.190]
And remembering to know you might not have heard it,
[00:24:41.360]
but remembering my much earlier points
[00:24:43.650]
about matching this where possible
[00:24:46.010]
to the drill hole complicit lens.
[00:24:48.500]
So that’s why I’m sort of picking the six here.
[00:24:53.500]
There’s also the ability in this tab to clump output values,
[00:24:59.740]
the default assumption is that
[00:25:02.610]
nothing should fall low zero,
[00:25:04.320]
which is why we’re seeing that clump,
[00:25:07.151]
but you can always change that if need be likewise,
[00:25:11.440]
you can set different lower,
[00:25:14.710]
and indeed upper bounds in the value transform tab
[00:25:19.280]
to cap any values you may deem too low or too high,
[00:25:23.360]
but I’m not going to focus on that for now.
[00:25:28.110]
So let me just let that run
[00:25:37.030]
and again, in the magic of eight forklifts,
[00:25:42.510]
so have a look at, so what that has produced,
[00:25:46.940]
and again, we’re starting to see something visually
[00:25:50.630]
that perhaps makes more sense.
[00:25:52.710]
That makes more sense to us and to our gold boundaries.
[00:25:58.990]
Now, if I want to see more connectivity
[00:26:03.010]
between my drill holes, then aside from the base range,
[00:26:07.550]
I could use a trend for this.
[00:26:09.630]
Also the central shear zone, for example,
[00:26:15.720]
is no doubt playing a dominant structural control
[00:26:21.870]
on my mineralization.
[00:26:23.750]
Starting to see a lot of our high grades,
[00:26:25.490]
sort of following some sort of trend here.
[00:26:29.370]
So applying a structural trend should help
[00:26:32.400]
to account for any changes in the strength
[00:26:36.409]
and direction of continuity along this ridge.
[00:26:41.330]
I’ve already defined a structural trends for the shear zone.
[00:26:46.230]
I sort of put it as something like this for now.
[00:26:51.130]
However we could have caused always update this as need be.
[00:26:55.410]
Either way, this is a typical step to take.
[00:26:58.640]
Given that nature is very rarely isotropic.
[00:27:03.530]
So let me go and apply this structural trends to my model
[00:27:11.960]
and which I can do from my trend tab.
[00:27:17.790]
And I’ve only got the one in my project at the moment.
[00:27:20.220]
So it’s just that stretch or trend,
[00:27:25.240]
and let’s just let that run.
[00:27:34.000]
And again, in a fantastic here’s one I prepared earlier,
[00:27:43.430]
we can see what that has done
[00:27:45.370]
this definitely you can see how that trend has changed
[00:27:49.870]
there is weighting of points in space
[00:27:52.780]
to define that continuity along that ridge.
[00:27:57.550]
So though powerful and usually they’ll be applicable
[00:28:01.560]
certainly in our sort of metallic gold
[00:28:05.776]
or indeed any deposit.
[00:28:09.970]
So now that we’ve sort of run to this point,
[00:28:14.870]
it’s likely at this stage
[00:28:17.030]
that we’re going to want to hone in our mineralize domains.
[00:28:21.590]
I spoke earlier about the early diorites unit
[00:28:27.780]
having our containing our highest mean gold grade.
[00:28:31.650]
So let’s come full circle and create a numeric model
[00:28:36.439]
for just this for you also.
[00:28:41.430]
So let me clear the scene and let me cheat
[00:28:48.370]
by copying my last model
[00:28:51.640]
with all of its parameters into the new one.
[00:29:05.230]
And now we can go into that copy of our model
[00:29:11.880]
and start to apply reasonable premises here as well.
[00:29:16.750]
Now, the first thing we’re going to want to do is of course,
[00:29:20.060]
set some boundary at the moment
[00:29:21.470]
it’s just the exact same as the last one,
[00:29:25.900]
which is the extent of my geological model.
[00:29:30.760]
Say, let’s go in and set a new lateral extent.
[00:29:34.830]
And what I’m going to do is use the volume
[00:29:37.971]
for my geological model as that extends.
[00:29:41.290]
Say from surface, and then under my geological models
[00:29:48.370]
under the output volumes,
[00:29:50.200]
I’m going to select that early diorite.
[00:29:57.230]
Now, whilst that’s running.
[00:29:59.920]
Remember that what that is going to do
[00:30:02.960]
is first of all, constrain your model
[00:30:06.710]
to that unit of interest.
[00:30:08.340]
So this is my early diorite.
[00:30:12.530]
And it’s also just only going to use the values
[00:30:22.910]
that refer to this unit,
[00:30:26.320]
what to do with that initial surface filter set up.
[00:30:29.700]
We then of course,
[00:30:30.533]
want to go and review our interplant settings here
[00:30:34.120]
to check that they’re still applicable.
[00:30:35.693]
Now that we’ve constrained our data to one domain.
[00:30:40.740]
Let me say, let me go back and double click into this model.
[00:30:46.240]
And let’s just double check once again,
[00:30:47.788]
that our interplant settings make sense.
[00:30:52.570]
It’s probably going to be more applicable in this case
[00:30:58.635]
that where we have an absence of data.
[00:31:01.290]
So again, on the outskirts, on the extents of our model,
[00:31:08.460]
that perhaps we’re going to want to revert
[00:31:10.730]
to the mean of the grade in the absence
[00:31:13.610]
of any other information.
[00:31:16.110]
In which case, the best thing that we can do
[00:31:19.030]
is simply to update the drift here to constant.
[00:31:23.890]
Hopefully you remember from that grid of points,
[00:31:26.570]
how that is always going to revert to the mean of the data.
[00:31:31.200]
I think for now,
[00:31:32.740]
I’m just going to leave every other setting the same,
[00:31:35.460]
but of course we could come in
[00:31:37.630]
and start to change any of these,
[00:31:41.270]
if we wish to see things a little bit differently,
[00:31:44.340]
but for now, it’s that drift that for me
[00:31:47.070]
is the most important.
[00:31:48.570]
So again, let me rerun and bring in a final output.
[00:32:02.240]
And if I just make these a little less transparent,
[00:32:06.520]
you can hopefully see now
[00:32:08.230]
that whereas before we had sort of that those waste grades
[00:32:14.230]
coming in on this sort of bottom corner,
[00:32:17.260]
we’re now reverting to what?
[00:32:19.500]
To something similar of the mean and I think in this case,
[00:32:23.330]
if I remember rightly the mean is yeah, 1.151.
[00:32:29.272]
So we would expect to be kind of up in that sort of darker,
[00:32:33.130]
darker yellow colors.
[00:32:38.020]
At this point,
[00:32:39.620]
I’m going to say that I’m reasonably happy
[00:32:42.230]
with the models I have.
[00:32:44.720]
I would, of course, want to interrogate these lot further.
[00:32:47.900]
Maybe make some manual edits
[00:32:50.502]
or double check some of the input data,
[00:32:53.578]
but for the purpose of this talk,
[00:32:55.490]
I hope that this has gone some way
[00:32:57.910]
in highlighting which interplant settings
[00:33:01.090]
will most effectively improve your numeric models.
[00:33:04.984]
I appreciate that this is a very extensive topic
[00:33:08.450]
to try and fit into a shorter amount of time.
[00:33:10.550]
Please do keep your questions coming in.
[00:33:14.090]
In the meantime,
[00:33:15.130]
I’m going to hand over to James now,
[00:33:19.030]
to run us through the indicator RBF interplant tool.
[00:33:24.550]
<v James>Thanks, Suzanna,</v>
[00:33:25.730]
bear with me two seconds and I’ll just share my screen.
[00:33:28.850]
So a lot of the stuff that Suzanna has run through
[00:33:32.350]
in those settings, we’re going to apply now
[00:33:34.830]
to the indicator numeric models
[00:33:38.520]
indicating numeric models are a tool
[00:33:41.530]
that is often underused or overlooked in preference
[00:33:46.220]
to the RBF models,
[00:33:47.810]
but indicator models can have a really valuable place
[00:33:51.480]
in anyone’s workflow.
[00:33:53.870]
So I’ve got the same project here,
[00:33:55.910]
but this time I’m going to be looking at
[00:33:58.050]
some of the copper grades that we have in the project.
[00:34:02.120]
If I go into a bit of analysis initially on my data,
[00:34:06.310]
I can see that when I look at the statistics for my copper,
[00:34:09.968]
there isn’t really a dominant trend to the geology
[00:34:16.350]
and where my copper is hosted is more of a disseminated
[00:34:19.879]
mineralization that is spread across multiple domains.
[00:34:24.480]
If I come in and have a look at the copper itself,
[00:34:27.600]
what I want to try and understand
[00:34:30.170]
is what it would be a good cutoff to apply
[00:34:34.740]
when I’m trying to use my indicator models
[00:34:37.580]
and this case here,
[00:34:38.650]
I’ve had a look at the histogram of the log,
[00:34:42.470]
and there’s many different ways
[00:34:44.380]
that you can approach cutoffs.
[00:34:46.954]
But in this case, I’m looking for breaks
[00:34:49.440]
in the natural distribution of the data.
[00:34:51.640]
And for today, I’m going to pick one around this area
[00:34:54.600]
where I see this kind of step change
[00:34:56.593]
in my grade distribution.
[00:34:59.410]
So at this point here,
[00:35:00.900]
I can see that I’m somewhere between 0.28 and 0.31% copper.
[00:35:06.770]
So for the purpose of the exercise,
[00:35:09.950]
we’ll walk through today we’ll use 0.3 as my cutoff.
[00:35:15.051]
So once I’ve had a look at my data and I have a better idea
[00:35:18.210]
of the kind of cutoffs
[00:35:19.660]
I want to use to identify mineralization,
[00:35:23.020]
I can come down to my numeric models folder,
[00:35:26.720]
right, click to create a new indicator.
[00:35:30.710]
I’ll be up in turbulent.
[00:35:34.186]
The layout to this is very similar to the numeric modeling.
[00:35:39.010]
Again, you can see,
[00:35:39.843]
that I can specify the values I want to use.
[00:35:43.050]
I can pick the boundaries I want to apply.
[00:35:45.090]
So for now, I’m just going to use the title project
[00:35:48.700]
in all my data.
[00:35:51.420]
And if I want to apply my query filters,
[00:35:53.730]
I can do that here as well.
[00:35:56.086]
A couple of other steps I need to do,
[00:35:58.670]
because this is an indicator interplant.
[00:36:00.820]
I need to apply a cutoff.
[00:36:02.730]
So what I’m doing here is
[00:36:03.590]
I’m trying to create a single surface
[00:36:05.960]
in closing grades above my cutoff of no 0.3.
[00:36:11.840]
And for the purpose of time,
[00:36:13.240]
I’m also going to just composite my data here.
[00:36:16.490]
So that it helps to run,
[00:36:18.710]
but it also helps to standardize my data.
[00:36:22.770]
So I’m going to set my composite length to four
[00:36:26.400]
and anywhere where I have residual links, less than one,
[00:36:29.240]
I’m going to distribute those equally back through my data
[00:36:33.950]
can give it a name to help me identify it.
[00:36:37.350]
And we’re going to come back
[00:36:38.500]
and talk about the ISO values in a minute.
[00:36:43.520]
So for now, I’m going to leave my ISO value
[00:36:45.338]
as a default of no 4.5, and then I can let that run.
[00:36:52.460]
So what leapfrog does with the indicators
[00:36:55.580]
is it will create two volumes.
[00:36:58.090]
It creates a volume that is considered inside my cutoff.
[00:37:02.150]
So if I expand my model down here,
[00:37:06.440]
we’ll see there’s two volumes as the output.
[00:37:08.800]
So there’s one that is above my console,
[00:37:11.960]
which is this volume.
[00:37:12.960]
And one that is below my cutoff,
[00:37:15.890]
which is the outside volume.
[00:37:19.775]
Now, at the moment,
[00:37:20.690]
those shapes are not particularly realistic.
[00:37:23.560]
Again, very similar to what we saw
[00:37:25.330]
with Suzanna’s explanation initially
[00:37:28.720]
because of the settings I’m using,
[00:37:30.100]
I’m getting these blow outs to the extent of my models.
[00:37:35.020]
The other thing we can have a quick look at
[00:37:36.388]
before we go and change any of the settings
[00:37:38.940]
is how leapfrog manages the data
[00:37:42.550]
that we’ve used in this indicator.
[00:37:45.050]
So it’s taken all of my copper values
[00:37:46.850]
and I’ve got my data here in my models.
[00:37:49.340]
So if I track that on,
[00:37:54.503]
just set that up so you can see it.
[00:37:57.690]
So initially from my copper values,
[00:38:01.310]
leapfrog will go and flag all of the data
[00:38:04.230]
as either being above or below my cutoff.
[00:38:07.060]
So here you can see my cutoff.
[00:38:09.270]
So everything above or below
[00:38:12.420]
it’s then going to give me a bit of an analysis
[00:38:15.870]
around the grouping of my data.
[00:38:18.130]
So here you can see that it looks at the grades
[00:38:22.570]
and it looks at the volumes it’s created,
[00:38:25.060]
and it will give me a summary of samples
[00:38:28.870]
that are above my cutoff and fall inside my volume,
[00:38:32.477]
but also samples that are below my cutoff
[00:38:35.080]
that are still included inside.
[00:38:37.510]
So we can see over here,
[00:38:38.530]
these green samples would be an example
[00:38:43.010]
where a sample is below my cutoff,
[00:38:45.977]
but has been included within that shell.
[00:38:49.360]
And essentially this is the equivalent
[00:38:51.200]
of things like dilution.
[00:38:52.110]
So we’ve got some internal dilution of these waste grades
[00:38:55.130]
and equally outside of my indicator volume,
[00:38:59.210]
I have some samples here that fall above my cutoff grade,
[00:39:04.290]
but because they’re just isolated samples,
[00:39:06.240]
they’ve been excluded from my volume.
[00:39:08.610]
So with that data and with that known information,
[00:39:13.800]
I then need to go back into my indicator
[00:39:16.160]
and have a look at the parameters and the settings
[00:39:18.671]
that I’ve used to see if they’ve been optimized
[00:39:20.910]
for the model I’m trying to build.
[00:39:23.820]
What we know with our settings,
[00:39:25.880]
and again, when we have a look at the inside volume,
[00:39:29.840]
if I come into my indicator here,
[00:39:32.327]
and go and have a look at the settings I’m using,
[00:39:35.726]
then I come to the interplant tab.
[00:39:39.260]
And for the reasons that Suzanna has already run through,
[00:39:42.740]
I know when modeling numeric data
[00:39:45.950]
and particularly any type of grade data,
[00:39:48.020]
I don’t want to be using a linear interplant.
[00:39:50.544]
I should be using my splital interplant.
[00:39:54.157]
The other thing I want to go and look at change in is that
[00:39:57.380]
I don’t have any constraints on my data at the moment.
[00:40:00.410]
So I’ve just used the model boundaries.
[00:40:02.950]
So I want to set my drift to known.
[00:40:06.410]
So again, there’s more of a conservative approach
[00:40:09.060]
as I’m moving away from data
[00:40:10.207]
and my assumption is my grade is reverting to zero.
[00:40:16.860]
We can also come and have a look at the volumes.
[00:40:20.090]
So currently our ISO value is five.
[00:40:23.020]
So we’ll come and talk about that in a second.
[00:40:25.340]
And the other good thing we can do here is
[00:40:26.920]
we can discard any small volumes.
[00:40:31.130]
So as an example of what that means,
[00:40:33.670]
if I take a section through my projects,
[00:40:40.000]
we can see that as we move through,
[00:40:41.440]
we get quite a few internal volumes
[00:40:44.910]
that aren’t really going to be of much use to us.
[00:40:47.920]
So I can filter these out based on a set cutoff.
[00:40:52.690]
So in this case,
[00:40:53.523]
I’m excluding everything less than 100,000 units,
[00:40:57.540]
and I can check my other settings to make sure
[00:40:59.450]
that everything else I’m doing,
[00:41:00.720]
maybe I used the typography is all set up to run how I want.
[00:41:05.480]
Again, what Suzanna talked about,
[00:41:07.018]
was there any time that you you’re modeling numeric data
[00:41:11.055]
best practice would be to typically use
[00:41:14.180]
some form of trend to your data
[00:41:16.560]
so I can apply the structural trend here
[00:41:18.460]
that’s Susanna had in hers, and I can let that one run.
[00:41:24.400]
Now, when that’s finished running,
[00:41:27.700]
I’ve got my examples here.
[00:41:31.800]
So if I load this one on,
[00:41:36.320]
I can see the outline here of my updated model
[00:41:39.240]
and how that’s changed the shape of my indicator volume.
[00:41:44.810]
So if we come back out of my section, put these back on,
[00:41:54.160]
I can see by applying those,
[00:41:56.410]
simply by applying those additional parameters.
[00:41:58.270]
So changing my interplant type from linear
[00:42:01.060]
to splital adding a drift of known
[00:42:03.510]
and my structural trend,
[00:42:06.830]
I’ve got a much more realistic shape now
[00:42:08.620]
of what is the potential volume of copper
[00:42:12.460]
greater than 0.3%.
[00:42:17.320]
Now, the next step into this
[00:42:19.250]
is to look at those ISO values
[00:42:20.930]
and what they actually do to my models,
[00:42:23.900]
the ISO value and if we come into the settings here,
[00:42:28.322]
so I’m just going to open up the sentence again.
[00:42:31.030]
You can see at the end, I can set an ISO value.
[00:42:34.140]
This is a probability of how many samples within my volume
[00:42:39.280]
are going to be above my cutoff.
[00:42:41.830]
So essentially if I took a sample
[00:42:44.380]
anywhere within this volume,
[00:42:46.230]
currently there is a 50% chance that,
[00:42:48.690]
that sample would be above my no 0.3% copper cutoff.
[00:42:53.871]
So by tweaking those indicator ISO values,
[00:42:58.860]
you actually can change the way the model is being built
[00:43:01.760]
based on a probability factor of how many,
[00:43:07.110]
what’s the chance of those samples
[00:43:08.940]
inside being above your cutoff.
[00:43:12.020]
So I’ve created two more with identical settings,
[00:43:15.190]
but just change the ISO value on each one.
[00:43:18.710]
If we step back into the model as a on a section,
[00:43:26.640]
so here we can see our model
[00:43:28.600]
which going to take the triangulations off.
[00:43:30.960]
So we’ve got the outline.
[00:43:33.320]
So currently with this volume,
[00:43:36.650]
what I’m saying is that I have a 50% chance
[00:43:39.497]
but if I take a sample, anywhere in here,
[00:43:41.780]
it is going to be above my cutoff
[00:43:44.810]
can also change my drill holes here to reflect that cutoff.
[00:43:49.810]
So you can see here, no 0.3% cutoff.
[00:43:52.460]
So you can see on my drilling samples
[00:43:55.470]
that are above and below.
[00:43:58.840]
If I go into my parameters
[00:44:01.000]
and I change my ISO value down to no 0.3.
[00:44:06.060]
So we can have a look at the inside value on this one,
[00:44:10.790]
what this is saying and I just made some,
[00:44:12.890]
so you can see it as well.
[00:44:13.810]
It’s basically, this is a volume
[00:44:15.930]
that is more of a prioritization around volume.
[00:44:18.830]
So this could be an example.
[00:44:20.130]
If you needed to produce a mean case and a max case
[00:44:23.677]
and a mid case,
[00:44:25.150]
then you could do this pretty quickly by using your cutoffs
[00:44:28.620]
and using your ISO values.
[00:44:31.620]
So drop in an ISO value down to 0.3
[00:44:35.120]
is essentially saying that there’s a 30% confidence
[00:44:38.130]
that if I take a sample inside this volume,
[00:44:41.240]
it will be above my cutoff.
[00:44:43.240]
So you can see that changing the ISO value
[00:44:45.960]
and keeping everything else the same
[00:44:47.300]
has given me a more optimistic volume
[00:44:50.640]
for my indicator shell.
[00:44:53.180]
Conversely, if I change my indicator ISO value to 0.7,
[00:44:58.780]
it’s going to be a more conservative shell.
[00:45:00.660]
So here, if I have a look at the inside volume in green,
[00:45:06.150]
and again, maybe just to help highlight
[00:45:07.740]
the differences with these.
[00:45:11.100]
So now this is my, exactly the same settings,
[00:45:15.220]
but applying a ISO value.
[00:45:18.260]
So a probability or a confidence of 0.7.
[00:45:22.070]
And again, just to, for you to review the notes.
[00:45:24.730]
So increase in my ISO value
[00:45:28.640]
will give me a more conservative case.
[00:45:31.720]
This will be prioritizing the metal content,
[00:45:34.500]
so less dilution.
[00:45:36.520]
And essentially I can look at those numbers and say,
[00:45:38.720]
I have a 70% confidence that any sample inside that volume
[00:45:42.700]
will be above my cutoff.
[00:45:47.020]
So I say it very quickly,
[00:45:48.520]
and particularly in the exploration field,
[00:45:50.610]
you can have a look at a resource or a volume.
[00:45:53.700]
And if you want to have a look at a bit of a range analysis
[00:45:56.300]
of the potential of your mineralization,
[00:45:58.500]
you can generate your cutoff and then change your ISO values
[00:46:03.340]
to give you an idea of how that can work.
[00:46:07.220]
Once you’ve built these,
[00:46:08.090]
you can also then come in and have a look at the,
[00:46:10.050]
it gives you a summary of your statistics.
[00:46:12.830]
So if we have a look here at the indicator at 0.3,
[00:46:16.040]
I look at the statistics of the indicator at 0.7,
[00:46:22.254]
we can go down to the volume.
[00:46:24.840]
So you can see the volume here
[00:46:27.610]
for my conservative ISO value at 0.7
[00:46:31.900]
is 431 million cubic meters,
[00:46:34.640]
as opposed to my optimistic shell, 545.
[00:46:40.330]
You can also see from the number of parts.
[00:46:42.350]
So the number of different volumes
[00:46:43.840]
that make up those indicators shells in the optimistic one,
[00:46:48.310]
I only have one large volume,
[00:46:50.930]
whereas the 0.7 is obviously a bit more complex
[00:46:54.520]
and has seven parts to it.
[00:46:57.314]
The last thing you can do
[00:46:59.190]
is you can have a look at the statistics.
[00:47:02.180]
So for example, the insight volume,
[00:47:04.720]
I can have a look at how many samples here
[00:47:06.970]
for below my cutoff.
[00:47:09.220]
So out of the what’s that 3,465 samples,
[00:47:15.260]
260 those are below my cutoff.
[00:47:19.200]
So you can kind of work out a, again,
[00:47:21.613]
a very rough dilution factor by dividing
[00:47:24.100]
the number of samples that fall inside your volume
[00:47:28.310]
by the total number of samples,
[00:47:29.610]
which would bring your answer around
[00:47:31.020]
in this case for the 0.3 around seven and a half percent.
[00:47:37.580]
So it’s just, this exercise is really just to highlight
[00:47:40.610]
some of the, again, the tools aren’t necessarily
[00:47:43.162]
used very frequently,
[00:47:45.200]
but can give you a really good understanding of your data
[00:47:48.930]
and help you to investigate the potential of your deposits
[00:47:53.200]
by using some of the settings in the indicator templates,
[00:47:58.669]
but ultimately coming back to the fundamentals
[00:48:02.620]
of building numeric models.
[00:48:04.870]
And that is to understand the type of interplant you use
[00:48:08.530]
and how that drift function can affect your models
[00:48:11.750]
as you move away from data.
[00:48:15.160]
So that’s probably a lot of content for you
[00:48:18.822]
to listen to in the space of an hour as always,
[00:48:23.500]
we really appreciate your attendance and your time.
[00:48:26.680]
We’ve also just popped up on the screen,
[00:48:28.580]
a number of different sources of training and information.
[00:48:33.810]
So if you want to go
[00:48:35.347]
and have a look at some more detailed workflows,
[00:48:38.440]
then I strongly recommend you look at the Seequent websites
[00:48:41.980]
or our YouTube channels.
[00:48:43.500]
And also in the, MySeequent page,
[00:48:45.930]
we now have all of the online content
[00:48:47.860]
and learning available there.
[00:48:50.110]
As always we’re available through the support requests.
[00:48:52.660]
So drop us an email
[00:48:54.057]
and if you want to get into some more detailed workflows
[00:48:57.820]
and how that can benefit your operations and your sites,
[00:49:01.830]
then let us know as well.
[00:49:03.570]
We’re always happy to support
[00:49:05.420]
via project assistance and training.
[00:49:08.900]
So that pretty much brings us to the end of the session
[00:49:13.290]
to the top of the hour as well.
[00:49:14.480]
So again, thanks to you for attending,
[00:49:17.780]
thanks to Suzanna and Andre for putting this together
[00:49:21.940]
and running the session.
[00:49:23.500]
And we’ll look to put another one
[00:49:25.700]
of these together again in the new year.
[00:49:27.690]
So hopefully we’ll see you all there.
[00:49:30.630]
Thanks everybody and have a good day.